
Remarkable leaps in brain–computer interface technology that could restore more naturalistic communication to people living with paralysis have been described in two recent papers in Nature. Both experimental systems have shown the ability to decode brain activity into speech faster, more accurately and with a larger vocabulary than existing alternatives.
Neurosurgeon Edward Chang of the University of California, San Francisco (UCSF) has been working on brain–computer interface technologies for more than a decade. In previous work, his team demonstrated that it was possible, using an implant, to decode into text the brain signals of a 30-year-man who had experienced a brainstem stroke 15 years earlier.
Their latest study has gone a step further, realising a neuroprosthesis that can not only translate brain activity into the full richness of speech, but also the facial movements that would accompany such conversation. Together, these two world-first accomplishments have allowed a woman with severe paralysis – also the result of a brainstem stroke – to talk via a digital avatar. The speech is synthesized via an algorithm, and personalized to sound like she used to, based on a recording from before her stroke.
“Our goal is to restore a full, embodied way of communication, which is really the most natural way for us to talk with others. These advancements bring us much closer to making this a real solution for patients,” says Chang in a press statement.
The researchers’ device takes the form of a paper-thin rectangle of 253 electrodes that they implanted over the region of the patient’s brain that is involved in speech. These electrodes intercept the neural signals that – had it not been for the stroke – would have activated muscles in her face, jaw, larynx and tongue.
The impulses were interpreted via deep-learning models that were trained over the course of several weeks by asking the patient to repeat different phrases from a 1024-word vocabulary. Rather than learning individual words, the systems instead operated on phonemes – the smaller subunits of speech analogous to letters in the written word.
The team found that the AI system only needed to learn 39 phonemes to decipher any word in English – allowing it to operate at 78 words-per-minute (wpm), a significant improvement over alternatives like eye-gaze systems (typically, people tend to speak at around 110–150 wpm; eye-gaze devices tend to enable only 5–15 wpm.)
The system racked up five times fewer errors than the previous state-of-the-art interface at decoding speech – with only a 4.9% word error rate when decoding sentences from a 50-phrase set – although this increased to a 25% error rate with a large vocabulary of over 1000 words. When using the synthetic voice output, a word error rate of 28% was encountered using a set of 529 phrases.
First author Sean Metzger, a bioengineer at UCSF, says: “The accuracy, speed and vocabulary are crucial. It’s what gives a user the potential, in time, to communicate almost as fast as we do, and to have much more naturalistic and normal conversations.”
In fact, the researchers note, the digital avatar can move its jaw, lips and tongue and reproduce a variety of expressions, including happiness, sadness and surprise. “When the subject first used this system to speak and move the avatar’s face in tandem, I knew that this was going to be something that would have a real impact,” says UCSF grad student Kaylo Littlejohn.
At present, the patient needs to be directly connected to the brain–computer interface. For the future, however, the team is working to develop a wireless version.
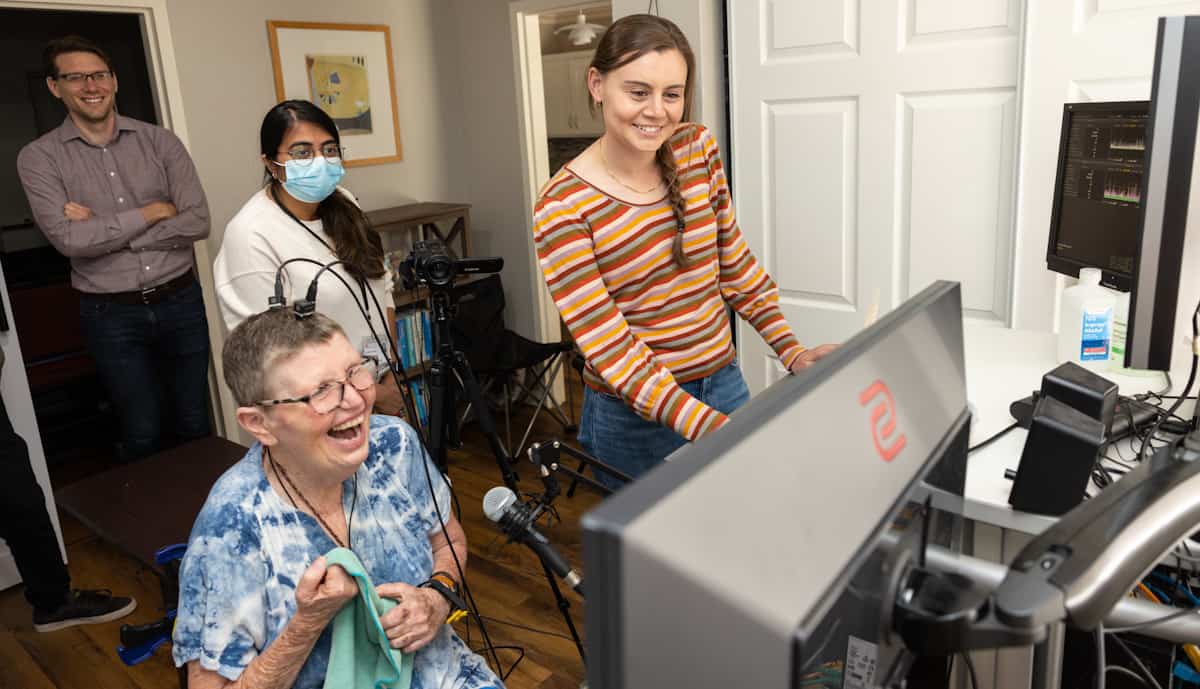
While Chang and his team decoded speech from a large number of cells across the entire speech cortex using a large array of electrodes, neurosurgeon Jaimie Henderson of Stanford University and his colleagues took a different approach — attaching just four tiny sensors, each containing a square array of 64 electrodes, into two speech-related brain regions in a patient with amyotrophic lateral sclerosis (ALS).
The recipient is Pat Bennett, a former HR director who was diagnosed with the progressive neurodegenerative disease in 2012. While ALS often first manifests in the body’s periphery as a result of deterioration in the spinal cord, for Bennett the condition began in her brain stem, leaving her still able to perform many tasks but unable to use the muscles of her lips, tongue, larynx and jaws to speak clearly.

Brain–computer interfaces: tailoring neurotechnology to improve patients’ lives
Four months and 25 four-hour AI-training sessions later, Bennett’s intended speech is converted into words on a computer screen at a rate of 62 wpm. When restricted to a 50-word vocabulary, the system’s error rate was 9.1%, increasing to only 23.8% with a comprehensive vocabulary of 125,000 words.
“We’ve shown you can decode intended speech by recording activity from a very small area on the brain’s surface,” Henderson concludes.
Sumner Norman – a biological engineer at the California Institute of Technology who was not involved in the two studies – tells Physics World that these advances are “a tour de force in technical and clinical excellence”. He added that both studies are a “wonderful demonstration” of how neurotechnologies can restore function capabilities for the impaired.