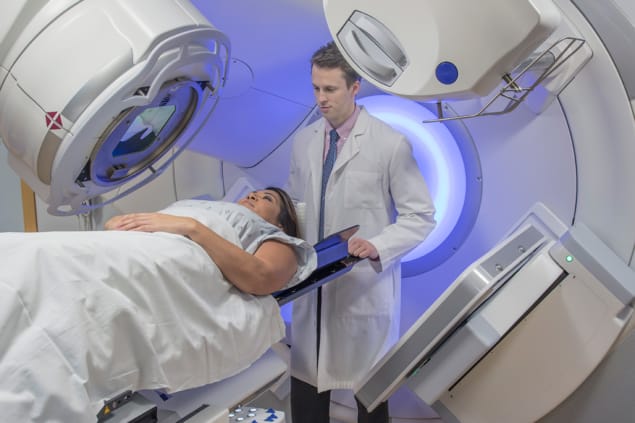
Online adaptive radiation therapy (ART) allows a treatment to be changed, or adapted, in response to additional information, such as weight loss or changes in tumour volume, gathered about a patient at the time of treatment. Adapting treatment plans in this way could improve patient outcomes – treatments delivered without adjustments might underdose targets or overdose organs-at-risk (OARs).
Since imaging for ART occurs while a patient is lying on the treatment couch, the acquired images must be contoured quickly and accurately. The low soft-tissue contrast on cone-beam CT (CBCT) images acquired during online ART, however, can make it challenging to delineate different structures. There’s also limited availability of “gold standard” contours for training deep-learning models.
A new framework addresses some of the challenges in segmenting CBCT images for online ART by using a deep-learning model to refine contours registered to a planning CT. The framework, developed by researchers at the University of Texas Southwestern Medical Center and Stanford University, is the first to apply a registration-based deep-learning segmentation model to segment OARs in head-and-neck cancers (at least one previous study has incorporated registration information to segmentation in thoracic cancers).
“As we are in an era of developing data-driven models rather than conventional analytical models, prior knowledge is critical. In radiotherapy clinics, there is abundant prior information. Utilizing this prior, existing information well is a direction for fast accurate segmentation and planning models development in radiotherapy,” says senior author Xuejun Gu, an associate professor of radiation oncology at Stanford University.
Registration-guided deep-learning segmentation framework
Image registration is the first of two components in the framework. The registration algorithm generates contours propagated from planning contours by registering the planning CT to the online CBCT using rigid or deformable registration approaches. The resulting contours for each OAR are input into the deep-learning model as binary masks. The second component of the framework is deep learning-based segmentation. The model outputs eight channels of probability masks consisting of OARs and “background” (i.e., everything that isn’t an OAR). The model is optimized by minimizing the volumetric soft Dice loss function.
Gu’s team tested the framework on an in-house head-and-neck cancer dataset consisting of 37 patients treated at a single institution. All CBCT images were acquired on a Varian TrueBeam onboard imaging system using the same machine settings, and all CBCT contours were delineated by the same physician. Any given patient may not have had a complete set of OARs due to surgical resection or tumour encroachment. It took the deep-learning model less than one second to generate final segmentations of the OARs when provided with registered CBCT contours.
Compared with registration or deep learning alone, the registration-guided deep-learning segmentation framework achieved more accurate segmentation as measured by distance-averaged metrics. The framework also appears to be less susceptible to image artefacts, such as streaking from dental implants.
Early stages are promising
The researchers claim that their framework, in addition to taking advantage of patient-specific positional information and population-based knowledge of organ boundaries, is stable even with limited training data.
“This study is significant,” says Gu. “First, it is a general framework. Second, introducing a patient-specific segmentation concept not only alleviates the data-demanding requirement of training deep-learning models but also improves segmentation accuracy, as the model is guided by patient-specific information.”
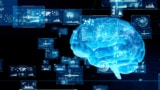
Machine learning makes its mark on medical imaging and therapy
The researchers acknowledge the obstacles they face going forward. Data curation is an ever-present challenge, as manually drawn contours are required for cross-validation. They are conducting additional robustness tests and generalizability tests to see how the model performs across institutions. They are also planning a systematic prospective study. And, as the quality of CBCT images and contouring protocols may vary across institutions, the researchers recommend that each institution commission its own model.
“The proposed deep learning-guided registration framework will enlighten researchers to develop models that incorporate prior knowledge,” Gu says. “We hope the impact of the study is beyond research, meaning the trained model can be translated into the clinic to assist patient treatment.”
This study was published in Medical Physics.
AI in Medical Physics Week is supported by Sun Nuclear, a manufacturer of patient safety solutions for radiation therapy and diagnostic imaging centres. Visit www.sunnuclear.com to find out more.